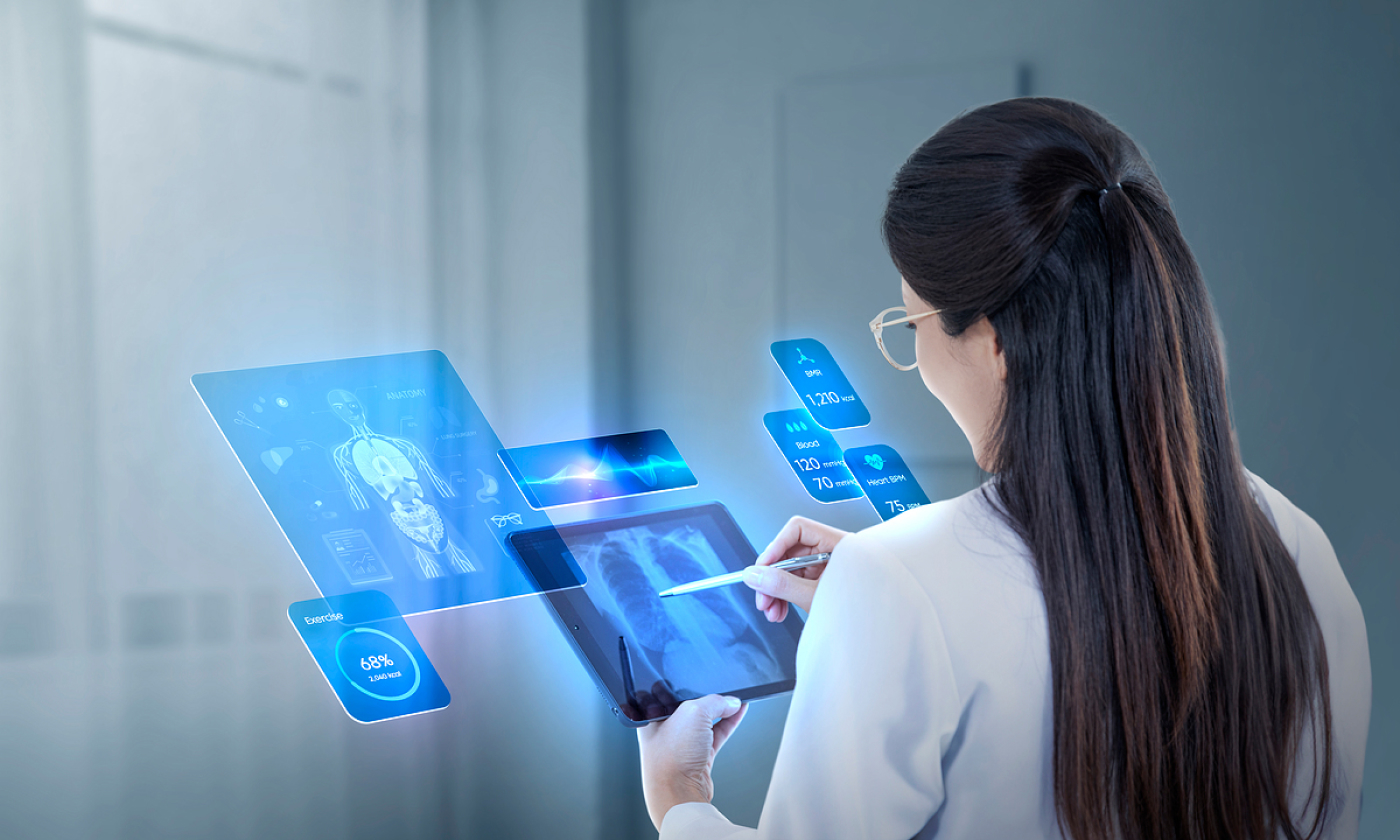
A research team led by Professor Kwang-Hyun Cho of the Department of Bio and Brain Engineering at Korea University of Science and Technology (KAIST) has developed a new concept of 'Gray Box' technology that can simultaneously predict drug responses and analyze mechanisms of cancer cells by combining AI and systems biology.
Despite research on cancer treatment, the number one cause of death in Korea, there are inherent limitations to applying targeted treatment using only traditional experimental biology approaches, as each patient has different genetic mutations that cause cancer and the resulting genetic network changes. On the other hand, using so-called black-box AI technologies such as deep learning can replace experiments and predict drug responses through data learning. Still, the biological basis cannot be explained, making it difficult to rely on the results.
Professor Kwang-Hyun Cho's research team came up with the so-called 'Gray Box' technology to overcome the limitations of both technologies simultaneously by combining deep learning, which is called a black box because it shows high prediction performance but its basis is unknown, and systems biology technology, which is called a white box because it has limitations in prediction performance but can provide a detailed basis for the prediction results.
The research team first established a general framework model that can be used to predict drug responses to various cancers and anticancer drugs by building a molecular regulatory network model by integrating information on mutations in various cancers and target genes of anticancer drugs. In particular, a pan-cancer gene network was created centered on genes with frequent mutations in various cancers, and a sub-network composed of mutations and associated genes related to drug responses for each target anticancer drug was extracted to create a systems biology model for predicting drug responses.
The significance of this research achievement is developing a new drug response prediction technology that simultaneously achieves the high predictive power of AI technology, a black-box model, and the interpretive power of systems biology technology, a white-box model, through simulation model optimization through learning. In particular, it is a universally applicable drug response prediction source technology for cancer, a heterogeneous and complex network disease. Hence, it is expected to suggest various types of cancer and customized treatment strategies for patients through future technological advancement.
Professor Kwang-Hyun Cho said, “As a new convergence source technology that simultaneously possesses the high predictive power of AI technology and the excellent interpretive power of systems biology technology, it is expected to be useful in the new drug development industry through future advancement.”